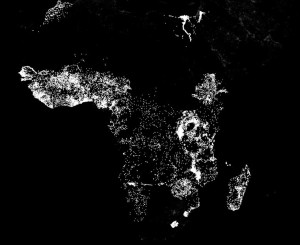
One of the biggest challenges in fighting poverty is the lack of reliable information. In order to aid the poor, agencies need to map the dimensions of distressed areas and identify the absence or presence of infrastructure and services. But in many of the poorest areas of the world such information is rare.
“There are very few data sets telling us what we need to know,” said Marshall Burke, an assistant professor in Stanford’s Department of Earth System Science and an FSE Senior Fellow at the Freeman Spogli Institute. “We have surveys of a limited number of households in some countries, but that’s about it. And conducting new surveys in hard-to-reach corners of the world, such as parts of sub-Saharan Africa, can be extremely time-consuming and expensive.
See more about this story @ news.stanford.edu